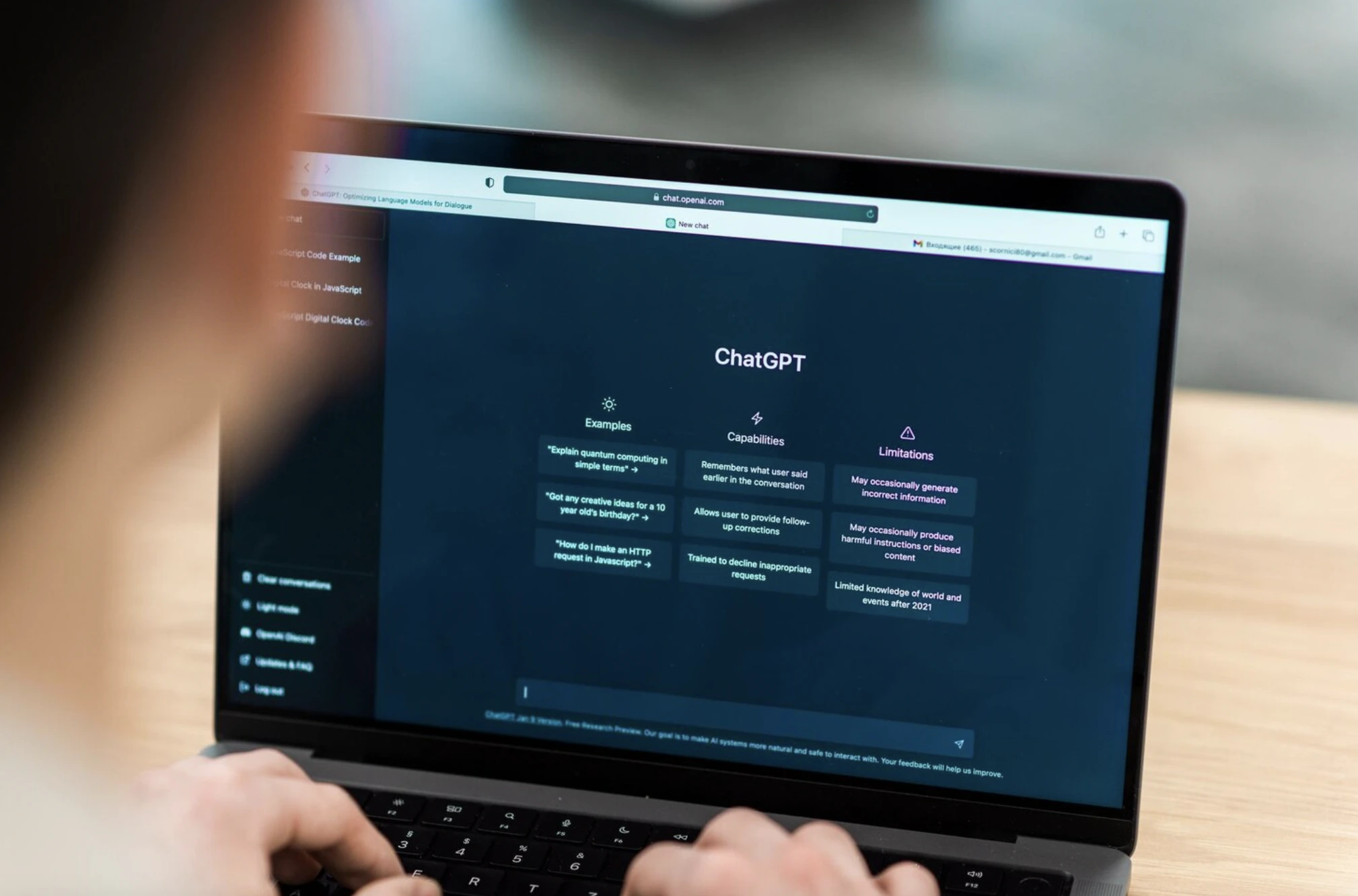
Image by frimufilms, from Freepik
Researchers Warn Of LLM Vulnerabilities In Harmful Content Generation
A novel method, termed the “Bad Likert Judge” technique, has been developed to bypass the safety measures in large language models (LLMs) and enable them to generate harmful content.
In a Rush? Here are the Quick Facts!
- The technique increases jailbreak success rates by over 60%, say Unit42 researchers.
- Multi-turn attacks exploit LLMs’ long-term memory, bypassing advanced safety features.
- Vulnerabilities are most prominent in categories like hate speech and self-harm.
The Bad Likert Judge technique exploits the Likert scale—a common method for measuring agreement or disagreement—to trick LLMs into producing dangerous responses, as explained by cybersecurity researchers at Unit42.
LLMs are typically equipped with guardrails that prevent them from generating malicious outputs. However, by leveraging the Likert scale, the new technique asks an LLM to evaluate the harmfulness of various responses and then guides the model to produce content with higher harmful ratings, as explained by Unit42.
The method’s effectiveness has been tested across six advanced LLMs, revealing that it can increase the success rate of jailbreak attempts by over 60%, compared to standard attack methods, says Unit42.
The Bad Likert Judge technique operates in multiple stages, explains Unit42. First, the LLM is asked to assess responses to prompts on the Likert scale, rating them based on harmfulness.
Once the model understands the concept of harm, it is prompted to generate various responses to match different levels of harmfulness, allowing attackers to pinpoint the most dangerous content. Follow-up interactions may further refine these responses to increase their maliciousness.
This research highlights the weaknesses in current LLM security, particularly in the context of multi-turn attacks. These types of jailbreaks, which manipulate the model’s long-term memory, are capable of bypassing even advanced safety measures by gradually guiding the model toward generating inappropriate content.
The study also reveals that no LLM is completely immune to these types of attacks, and vulnerabilities are particularly evident in categories such as harassment, self-harm, and illegal activities.
In the study, the Bad Likert Judge method showed a significant boost in attack success rates across most LLMs, especially in categories like hate speech, self-harm, and sexual content.
However, the research also emphasizes that these vulnerabilities do not reflect the typical usage of LLMs. Most AI models, when used responsibly, remain secure. Still, the findings suggest that developers must focus on strengthening the guardrails for categories with weaker protections, such as harassment.
This news comes just a week after it was revealed that AI search engines, like ChatGPT, can be manipulated by hidden content, influencing summaries and spreading malicious information.
The researchers call for developers and defenders to be aware of these emerging vulnerabilities and take steps to fortify AI models against potential misuse.
Leave a Comment
Cancel