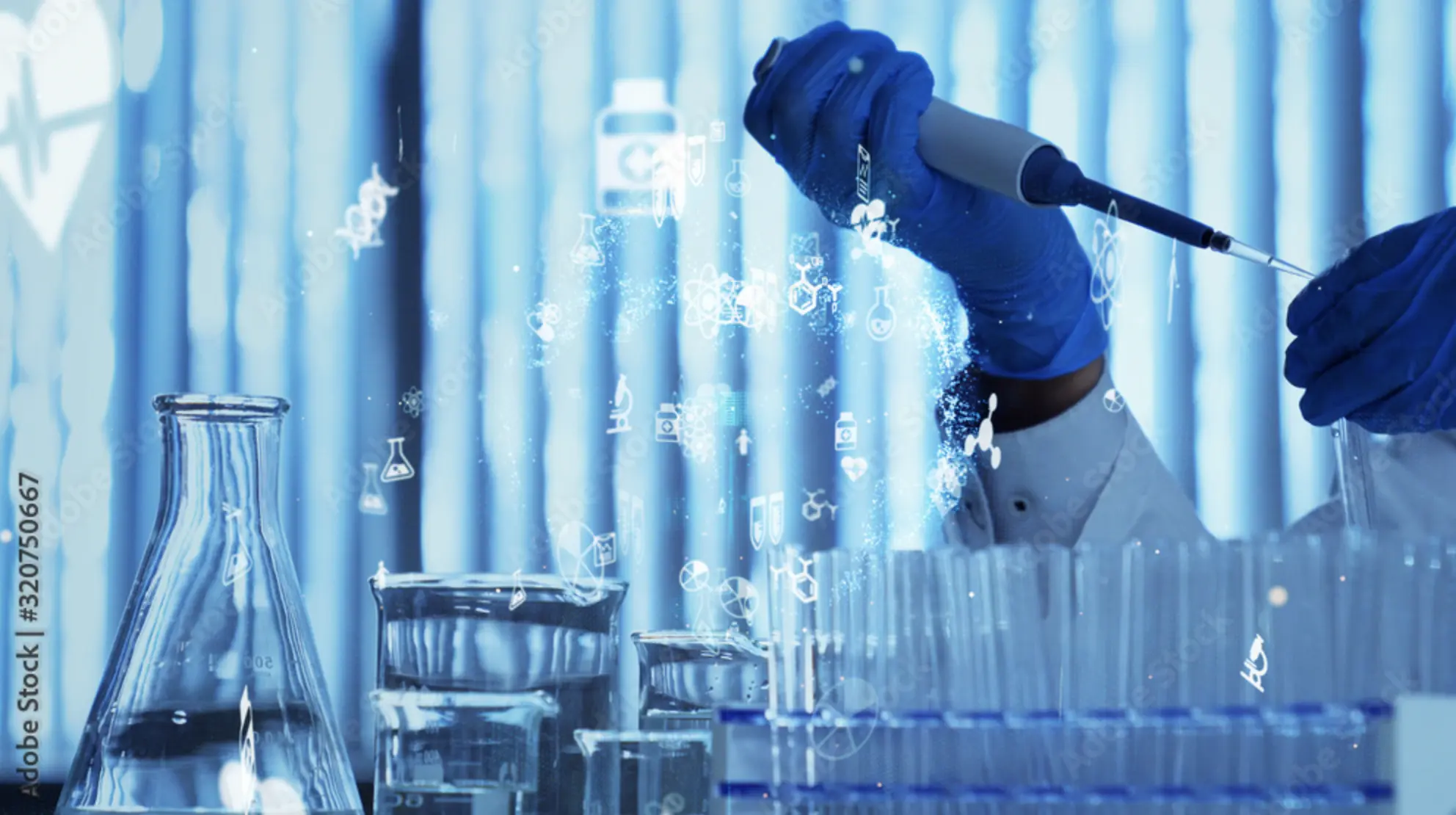
Image by metamorworks, from Adobe Stock
Researchers Aim To Improve AI’s Role In Chemistry
Researchers are working to understand how AI makes decisions to enhance its use in chemistry, especially drug development. New research on this topic will be presented at the American Chemical Society’s Fall meeting, from August 18 to 22. The meeting will feature over 10,000 presentations on various scientific topics.
Today, the American Chemical Society announced in a press release that researchers will present findings on using Explainable AI (XAI) to understand how AI make decisions in chemistry. By demystifying these complex systems, experts aim to increase trust and improve AI’s effectiveness.
Rebecca Davis, a chemistry professor, explains that current AI models are often seen as “black boxes,” with their decision-making processes concealed. She states, “If we can come up with models that help provide some insight into how AI makes its decisions, it could potentially make scientists more comfortable with these methodologies.”
Additionally, XAI can provide valuable information to refine AI programming and enhance its performance in the field. “I want to use XAI to understand what information we need to teach computers about chemistry,” says Hunter Sturm, a graduate student in Davis’ lab presenting the work at the meeting.
The researchers began by feeding databases of known drug molecules into an AI model designed to predict a compound’s biological activity. To understand the model’s decision-making process, they employed an XAI model developed by collaborator Pascal Friederich at Germany’s Karlsruhe Institute of Technology.
This allowed them to identify the specific molecular features influencing the AI’s predictions. By analyzing these factors, Davis and Sturm gained insights into the AI’s criteria for categorizing molecules and determining their potential as drug candidates.
Preliminary findings suggest that XAI can detect things humans might overlook, since it can analyze far more variables at once.
For example, when analyzing penicillin molecules, the AI discovered something unexpected. Scientists generally believe the core structure of penicillin is crucial for its antibiotic properties. However, Davis explained that the XAI identified the parts attached to this core as the key factor in determining the molecule’s antibiotic activity. She states that this could explain why some modified versions of penicillin with the same core structure are less effective.
As a next step, the researchers plan to collaborate with a microbiology lab to create and test potential antibiotic compounds identified by the improved AI models. Their ultimate goal is to use AI to develop new and more effective antibiotics that can combat the growing threat of antibiotic resistance.
In a presentation, Davis states, “Machine learning now offers us the opportunity to do this on a much larger scale, where we’re likely to see trends that we have never seen in chemical behavior and will allow us to create molecules and materials at a much faster rate than we ever have in history.’’
As AI continues to evolve, transparency and comprehension are essential for fostering public as well as scientific trust. By unraveling the complexities of AI, scientists are making progress towards harnessing its full potential for societal benefit.
Leave a Comment
Cancel