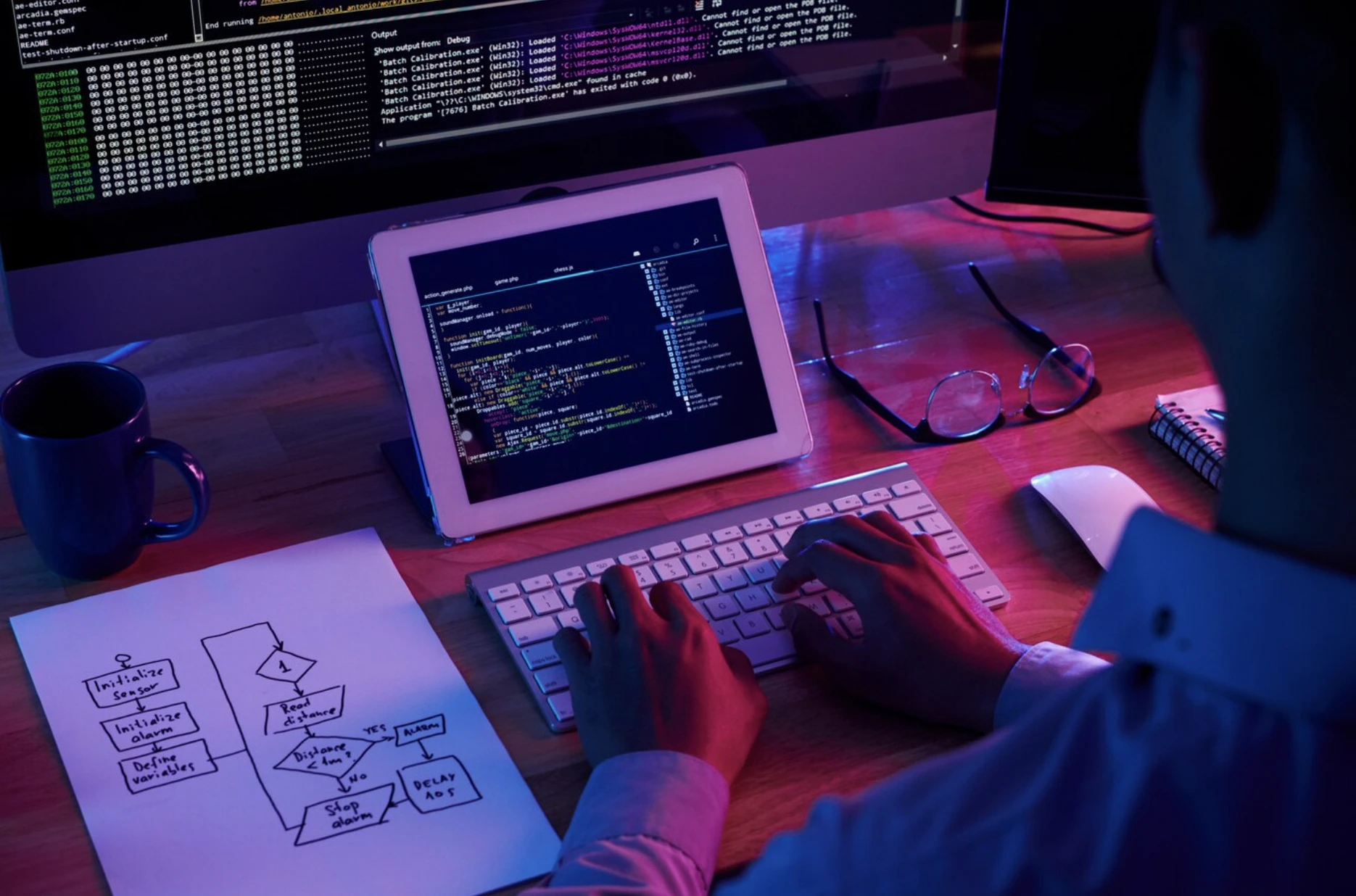
Image by pressfotos, from Freepik
New MIT Algorithm Boosts AI Decision-Making Efficiency By Up to 50 Times
MIT researchers developed an efficient AI training algorithm, boosting performance by selecting the best tasks, improving decision-making and reducing training costs.
In a Rush? Here are the Quick Facts!
- Model-Based Transfer Learning (MBTL) improves performance while reducing data and computational needs.
- MBTL was five to 50 times more efficient than traditional reinforcement learning methods.
- The researchers plan to extend MBTL for more complex real-world problems.
MIT researchers have introduced a new algorithm designed to make AI decision-making models more efficient, especially for complex tasks like controlling traffic in cities.
The algorithm improves upon traditional reinforcement learning, which often struggles with variability across different tasks.
The MIT press release explains that for example, an AI model trained to control traffic at one intersection might fail when applied to others with different traffic patterns, lane numbers, or speed limits.
The new approach, known as Model-Based Transfer Learning (MBTL), strategically selects a subset of tasks to train the AI agent, focusing on those that will provide the most significant improvements in performance.
By narrowing the training focus, this method reduces the data and computational resources required while boosting the efficiency of the learning process, says MIT.
The team’s research, which will be presented at the Conference on Neural Information Processing Systems, demonstrated that MBTL was between five and 50 times more efficient than standard methods.
“We were able to see incredible performance improvements, with a very simple algorithm, by thinking outside the box,” said Cathy Wu, senior author and associate professor at MIT.
“An algorithm that is not very complicated stands a better chance of being adopted by the community because it is easier to implement and easier for others to understand.”
Typically, AI models for tasks like traffic control are trained in one of two ways: either using data from all tasks, or training separate models for each task.
MIT explains that both methods have drawbacks—training separate models requires huge amounts of data, while training on all tasks often leads to subpar performance.
The researchers’ method finds a middle ground, training an algorithm on a smaller subset of tasks that are strategically selected to maximize performance across all tasks.
MBTL uses zero-shot transfer learning, a concept where a model trained on one task is applied to similar tasks without additional retraining.
MIT explains that this method estimates how well the model will perform on tasks it hasn’t been directly trained for, thus selecting tasks that will improve overall generalization.
“With a 50x efficiency boost, the MBTL algorithm could train on just two tasks and achieve the same performance as a standard method which uses data from 100 tasks,” Wu explained.
This approach significantly reduces the amount of training data required, improving both the speed and cost-effectiveness of developing AI models for complex decision-making, according to MIT.
Looking ahead, the team plans to refine the MBTL method for more complicated systems and real-world applications, such as next-generation mobility systems.
Leave a Comment
Cancel