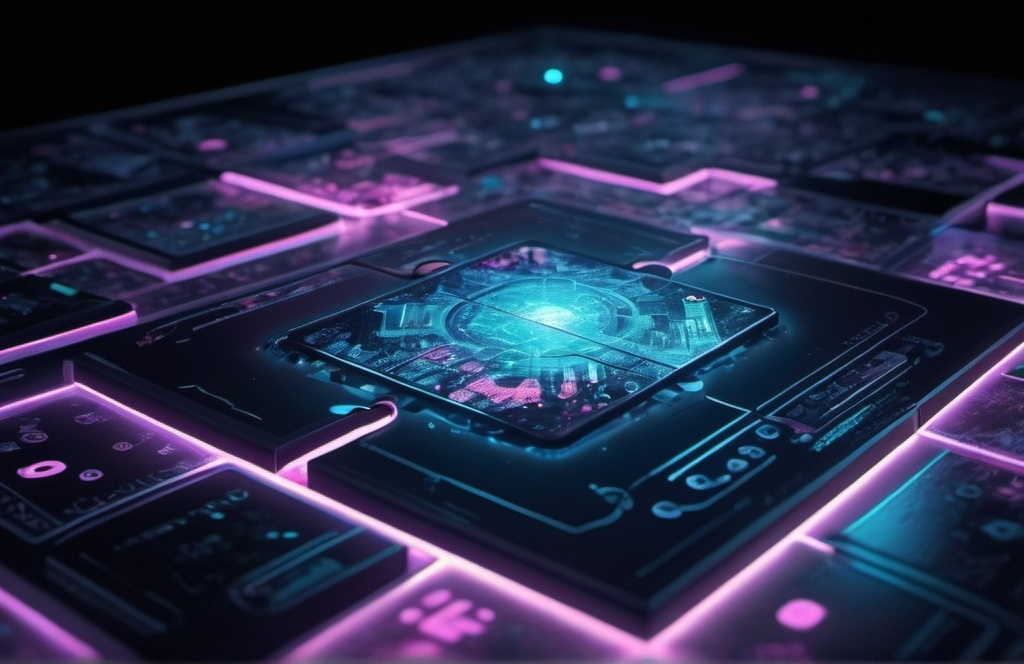
Image created with Openart.ai
MIT Reveals That LLMs May Develop Their Own Understanding of Reality
Researchers at MIT have found that large language models (LLMs) can create their own internal representations of reality. Training an LLM on puzzles revealed that the model developed an understanding of the puzzle’s environment on its own, without explicit instruction. MIT News reported on the news yesterday.
To test this, researchers used Karel puzzles—tasks that involve giving instructions to a robot in a simulated environment to help solve them. After training the model on over 1 million such puzzles, they found that the LLM not only improved in generating correct instructions but also appeared to develop an internal simulation of the puzzle environment.
Charles Jin, the lead author of the study, explained, “At the start of these experiments, the language model generated random instructions that didn’t work. By the time we completed training, our language model generated correct instructions at a rate of 92.4 percent.”
This internal model, uncovered using a machine learning technique called “probing,” revealed an internal model of how the robot responded to instructions, suggesting a form of understanding beyond syntax.
The probe was designed merely to “look inside the brain of an LLM,” as Jin puts it, but there was a chance it might have influenced the model’s thinking.
Jin explains, “The probe is like a forensics analyst: You hand this pile of data to the analyst and say, ‘Here’s how the robot moves; now try and find the robot’s movements in the pile of data.’ The analyst later tells you that they know what’s going on with the robot in the pile of data.’’
Jin adds, “But what if the pile of data actually just encodes the raw instructions, and the analyst has figured out some clever way to extract the instructions and follow them accordingly? Then the language model hasn’t really learned what the instructions mean at all.”
To test this, the researchers carried out a “Bizarro World” experiment in which the meanings of instructions were reversed. In this scenario, the probe had difficulty interpreting the altered instructions, suggesting that the LLM had developed its own semantic understanding of the original instructions.
These results challenge the prevailing view that LLMs are merely sophisticated pattern-matching machines. Instead, it suggests that these models may be developing a deeper, more nuanced comprehension of language and the world it represents.
A study from the University of Bath earlier this week indicated that LLMs excel at language processing but struggle with independent skill acquisition. This reinforced the idea of LLM predictability. However, the MIT research offers a contrasting perspective.
Even though the MIT results seem promising, the researchers point out some limitations. Specifically, Jin acknowledges that they used a very simple programming language and a relatively small model to obtain their insights.
Leave a Comment
Cancel