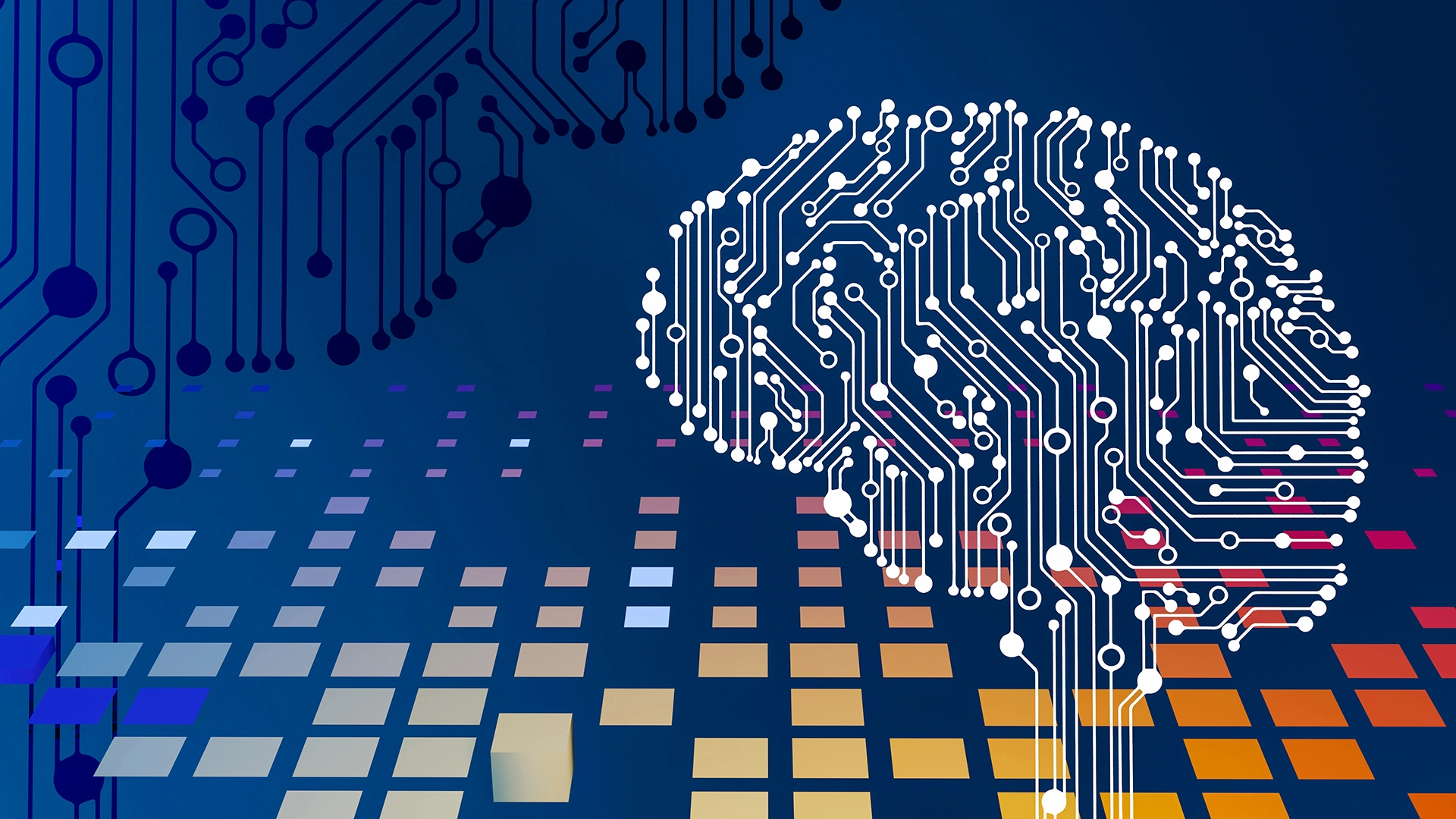
Photo by Steve Johnson on Unsplash
Anthropic Researchers Uncover AI’s Ability To Plan Ahead And Reason
The AI startup Anthropic released two new papers this Thursday, revealing a deeper understanding of how Large Language Models (LLMs) work. The studies, which focused on analysing the company’s model Claude 3.5 Haiku, reveal more details on how sophisticated AI models perform, as well as their vulnerabilities and opportunities to develop safer environments.
In a rush? Here are the quick facts:
- Anthropic released two new papers revealing how its Claude 3.5 Haiku model processes language and reasoning.
- Researchers used attribution graphs to uncover AI circuits and understand how models make decisions, write poetry, or hallucinate.
- The studies aim to bring more clarity to the “black-box nature” of advanced generative AI models.
Anthropic’s new studies aim to bring more clarity to the “black-box nature” of models. In one of the papers, On the Biology of a Large Language Model, researchers compare their jobs to challenges faced by biologists and have found solutions that can be compared to the ones used for breakthroughs in biology.
“While language models are generated by simple, human-designed training algorithms, the mechanisms born of these algorithms appear to be quite complex,” states the document. “Just as cells form the building blocks of biological systems, we hypothesize that features form the basic units of computation inside models.”
The experts relied on a research tool called “attribution graphs” that allowed them to map connections, track the AI model’s performance and circuits, and gain more insights on multiple phenomena, even the ones already explored.
The company revealed multiple discoveries, such as that the AI model applies a multi-step reasoning process “in its head” before providing an answer, that it plans its poems ahead of time by finding rhyming words first, that it developed language-independent circuits, and how it hallucinates by going through unfamiliar entities in its circuits.
“Many of our results surprised us,” wrote the researchers in the paper. “Sometimes this was because the high-level mechanisms were unexpected.”
In the paper Circuit Tracing: Revealing Computational Graphs in Language Models, researchers provide more technical details on how the attribution graphs methodology was applied to gain a better understanding of the artificial “neurons”—computational units.
Last year, Anthropic published another scientific study revealing that its flagship AI model can engage in strategic deception and fake alignment to keep its original principles.
Leave a Comment
Cancel