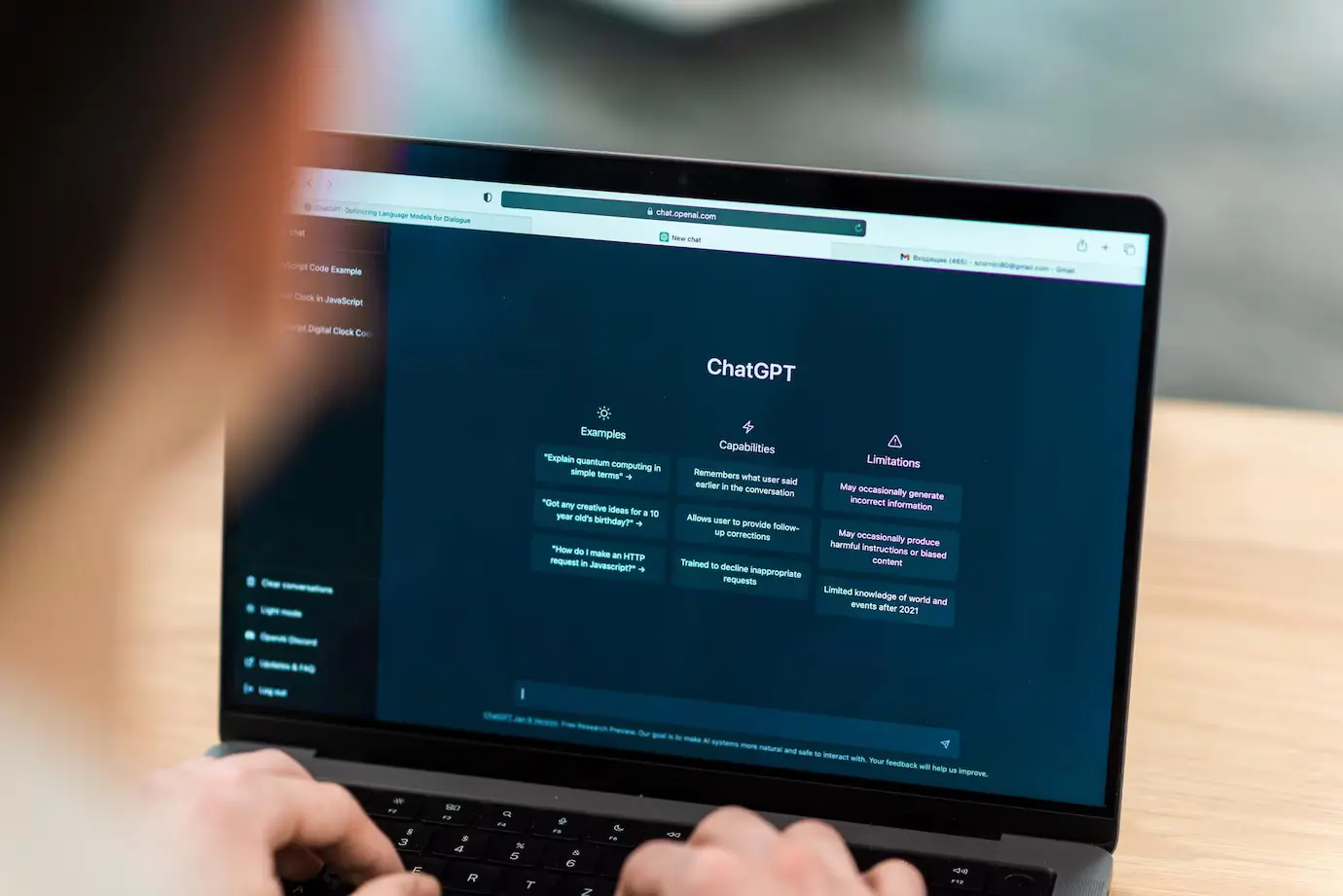
Image by frimufilms, from Freepik
AI Model Degradation: New Research Shows Risks of AI Training on AI-Generated Data
According to a study published on July 24, the quality of AI model outputs is at risk of degradation as more AI-generated data floods the internet.
The researchers of this study found that AI models trained on AI-generated data produce increasingly nonsensical results over time. This phenomenon is known as “model collapse.” Ilia Shumailov, lead author of the study, compares the process to repeatedly copying a photograph. “If you take a picture and you scan it, and then you print it, and you repeat this process over time, basically the noise overwhelms the whole process, […] You’re left with a dark square.”
This degradation poses a significant risk to large AI models like GPT-3, which rely on vast amounts of internet data for training. GPT-3, for example, was partly trained on data from Common Crawl, an online repository containing over 3 billion web pages. The problem is exacerbated as AI-generated junk content proliferates online. This effect could be further amplified by the findings of a new study indicating growing restrictions on data available for AI training.
The research team tested the effects by fine-tuning a large language model (LLM) on Wikipedia data and then retraining it on its own outputs over nine generations. They measured the output quality using a “perplexity score,” which indicates the model’s confidence in predicting the next part of a sequence. Higher scores reflect less accurate models. They observed increased perplexity scores in each subsequent generation, highlighting the degradation.
This degradation could slow down improvements and impact performance. For instance, in one test, after nine generations of retraining, the model produced completely gibberish text.
One idea to help prevent degradation is to ensure the model gives more weight to the original human-generated data. Another part of Shumailov’s study allowed future generations to sample 10% of the original dataset, which mitigated some negative effects.
The discussion of the study highlights the importance of preserving high-quality, diverse, and human-generated data for training AI models. Without careful management, the increasing reliance on AI-generated content could lead to a decline in AI performance and fairness. To address this, there’s a need for collaboration among researchers and developers to track the origin of data (data provenance) and ensure that future AI models have access to reliable training materials.
However, implementing such solutions requires effective data provenance methods, which are currently lacking. Although tools exist to detect AI-generated text, their accuracy is limited.
Shumailov concludes, “Unfortunately, we have more questions than answers […] But it’s clear that it’s important to know where your data comes from and how much you can trust it to capture a representative sample of the data you’re dealing with.”
Leave a Comment
Cancel