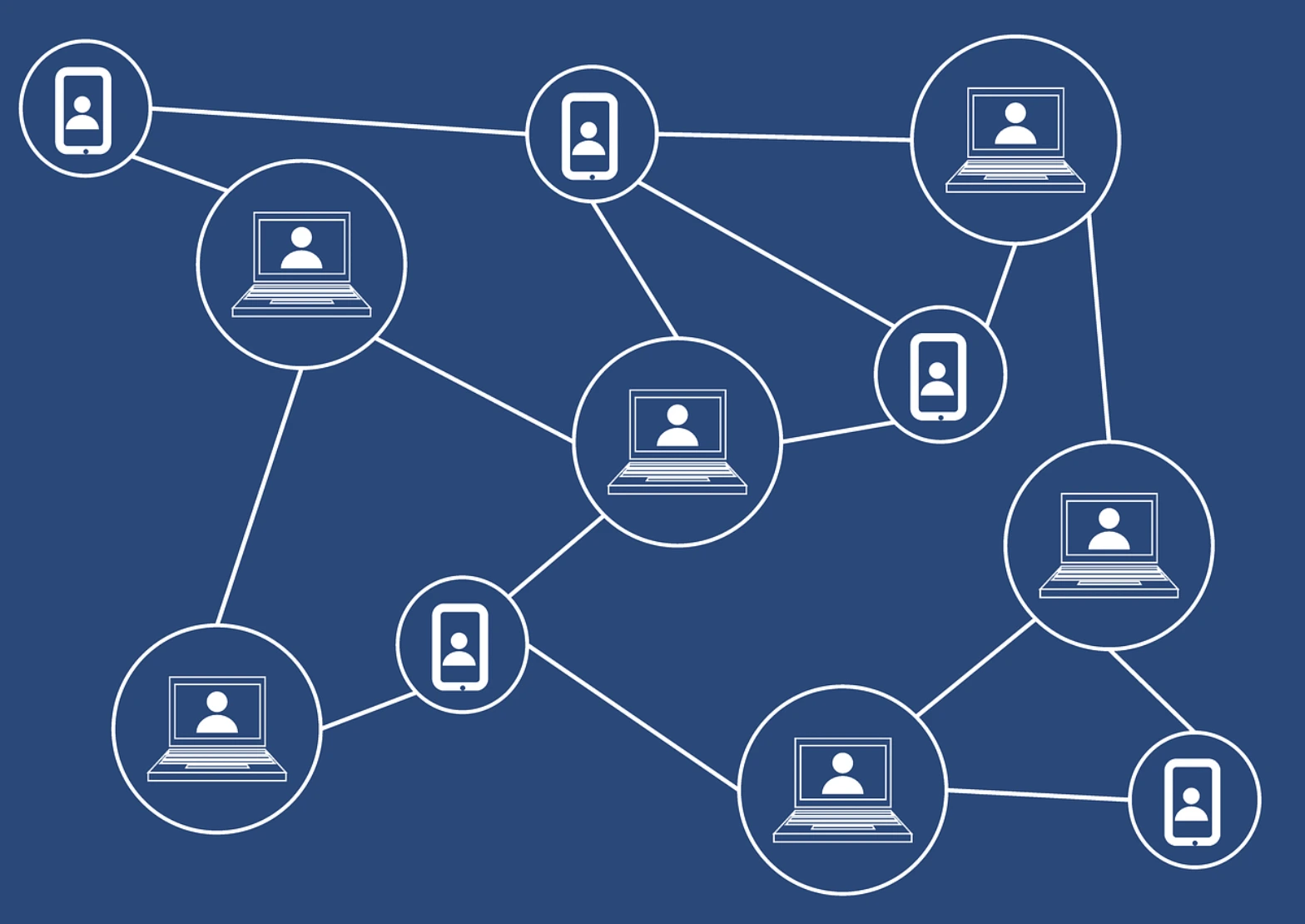
Image by Tumisu, from Pixabay
Researchers Introduce Blockchain Framework To Democratize Deep Reinforcement Learning
In a Rush? Here are the Quick Facts!
- Crowdsourced DRL framework enhances accessibility and training.
- Blockchain ensures transparency, security, and traceability.
- Decentralization lowers costs and democratizes DRL.
A team of researchers, led by Concordia University, announced yesterday a novel blockchain-based framework to make deep reinforcement learning (DRL) more accessible.
DRL, a branch of AI that combines deep learning and reinforcement learning, has proven valuable in industries such as gaming, robotics, healthcare, and finance. However, due to its complexity, it remains out of reach for many small businesses and individuals.
To bridge this gap, the researchers developed a crowdsourced DRL as a Service (DRLaaS) framework, which allows users to access DRL-related services, including model training and sharing.
This new framework enables users to tap into the expertise and computational capabilities of workers who can train DRL models on their behalf. Furthermore, users can benefit from pre-trained models shared by workers, which can then be customized through knowledge transfer methods.
Built on a Consortium Blockchain, this framework ensures transparency and traceability in the execution of tasks. The system employs smart contracts to manage task allocation, and models are stored using the InterPlanetary File System (IPFS) to maintain data integrity.
By using blockchain technology, the framework addresses concerns related to server failures and data tampering.
According to lead author Ahmed Alagha, the crowdsourcing aspect enhances accessibility, allowing more people to participate in developing DRL solutions.
“With this framework, anyone can sign up and build a history and profile. Based on their expertise, training and ratings, they can be allocated tasks that users are requesting,” said Alagha.
The authors claim that the system’s decentralization also reduces the risk of catastrophic failures and lowers the costs associated with training DRL models.
The authors state that by distributing computational efforts across multiple machines, the system offers resilience against server crashes or cyberattacks, a significant improvement over traditional centralized systems.
Co-author Jamal Bentahar, Alagha’s thesis supervisor, emphasized that this service democratizes access to DRL solutions.
“To train a DRL model, you need computational resources that are not available to everyone. You also need expertise. This framework offers both,” Bentahar noted.
The full details of this framework can be found in their research paper, published in Information Sciences, which highlights the framework’s design and its potential applications.
Leave a Comment
Cancel